The inner workings of deepfake technology
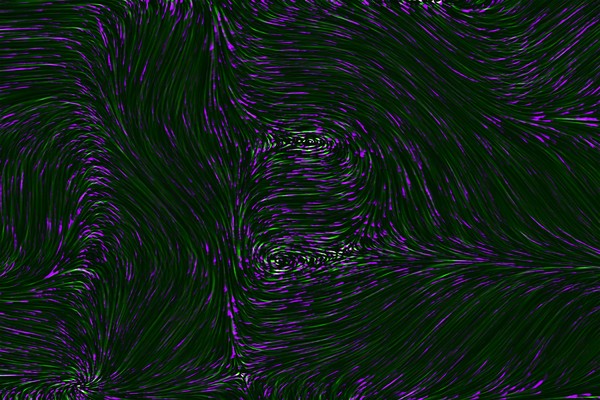
DEEPFAKES ARE a product of synthetic media[1] consumption. The term derives from “deep learning,” which is a self-learning algorithm designed to replicate the network of a human brain, and the word “fake.” In its simplest form, this technology allows the creation of falsified videos by attaching the face of a nonparticipating individual onto another body. The result is a variety of different content with the purpose of entertaining or stimulating responses from the public. While seeing a meteoric rise to popularity in recent years, deepfakes are far from harmless. They have been tied to illicit industries, such as pornography and fraud, and many have called for the regulation of deepfakes before further damages are inflicted.
The science behind deepfakes
Deepfakes consist of artificial intelligence (AI) machinery and facial recognition extensions that can filter through several images. The software of this technology differs from the more well-known computer-generated imagery (CGI) found in films and television. CGIs are computerized graphics, often created frame by frame, and are dependent on the humans behind the screen. Many consider it to be a sophisticated form of animation. Deepfakes, on the other hand, are generated without much human intervention, as it only requires competent software that can be trained to perform certain tasks autonomously. Deepfake Artificial Intelligence (AI) can synthesize facial features by studying up to millions of images, duplicate speech through pre-existing recordings, and imitate actions. The realism of a deepfake improves with the amount of information fed through the algorithm. In that regard, deepfake technology will develop with time, as its algorithm continues to acquire information from either a content creator or the cloud.
A common way to produce deepfakes is through an image generating architecture known as a variational auto-encoder (VAE). VAEs carry two encoders: one to “encode images into low-dimensional representations, and then [another to] decode those representations back into images[2].” They essentially filter through several shots of a person’s face and insert them onto each frame in a video. A more diverse range of images in terms of angles and lighting ensures a more convincing deepfake. Hence, apart from the larger incentive to create deepfakes for famous individuals, the abundance of images that can be found online makes celebrity deepfakes easier to create. Celebrities are not the only victims of deepfakes, however, with the scope of modern deepfakes expanding far beyond video content.
Alternative deepfakes
Although deepfakes are mostly understood as fabricated videos, there are various alternatives that can be created based on similar AI technology:
Audio deepfakes replicate the voice of a designated person to process words from text-to-speech (TTS). Audio cloning software already exists for the public with programs such as “Deep Voice,” presented in the 34th International Conference on Machine Learning, and technology comapny NVIDIA’s “Tacotron2.” An edited audio recording of former US President Donald Trump reciting a monologue from the Star Wars franchise used the latter program, and received over a million views on YouTube. Whilst TTS generators have existed for several years, the added AI component aids with creating authentic speech that is less artificial and more human-like in cadence. Its algorithm studies voice inflections and accents from a variety of different recordings, using the data to produce near-perfect results.
Textual deepfakes can also be produced at a simpler degree. It incorporates the technology found in chatbot software, or programs that conduct auto-generated human conversation. By highlighting keywords from its target’s messages, they can form appropriate responses while naturally navigating the conversation towards a desired topic. Textual deepfakes are arguably the most difficult to detect, as sophisticated AI mimic human-made indiscernible messages , which may cause problems for unassuming victims.
Effects of deepfake technology
One of the main culprits of deepfake technology’s infamy is its growing production of illicit pornography. The first notable case of X-rated deepfake content rose from an online forum called Reddit in 2017 when an anonymous user posted a series of pornographic material featuring female celebrities[3]. Since then, there has been an explosive rise of falsified pornography, with a 2019 study on deepfakes reporting that 96% of all deepfake content found on the internet was pornographic in nature[4]. The following year, another report revealed how there were 27,271 X-rated deepfake content found across 30 pornography websites on the internet[5]. A quarter of deepfake pornography reportedly features K-pop stars[6], with websites and encrypted chatrooms solely featuring deepfake pornography amass up to 100,000 users.
Fake news is another product of this technology. Millions of those unaware or unable to detect deepfakes may mistake deepfakes for fact. This exact situation occurred in March, 2022 amidst the ongoing Russo-Ukrainian War. A fabricated video of Ukrainian President Volodymyr Zelenskyy was released online and on cable, with him calling for the surrender of all Ukrainian citizens. Zelenskyy was quick to deny the video’s authenticity, but the damage was done amid high fear and tensions.
Solutions for illicit deepfakes
Some detection software, supported by either governments or private companies, are in the works to combat the rise of illicit deepfake content. These AI algorithms track deepfakes with a series of different methodologies, such as signal feature-based methods or physical/physiological-based methods[7]. The first method detects abnormalities within every frame of a video that may have been created during the fabrication process. The algorithms are programmed to recognize inhuman facial features, which includes warped faces and poor blending. Physical/physiological-based methods track behaviors usually lacking in deepfakes such as eye-blinking patterns, breathing, and natural twitches. Inconsistencies often prove that a video is not a deepfake, whilst perfectly synchronized patterns of movement reveal otherwise.
Parallel to the relative recentness of deepfake technology, deepfake detection technology is still in its nascent stage. An improvement in media literacy may be the only method to combat the deepfake epidemic whilst experts research accurate and dependable detection software. Spreading awareness on deepfakes can inform netizens[8] on the dangers of the technology. And, preventing the creation of illicit deepfakes by regulating deepfake editing applications may also impede the ongoing issue.
* * *
Deepfakes are an intriguing and entertaining product of technology that has caught the public’s attention. The algorithm behind such content reflects human ingenuity, but its harmless origins have devolved into a tool to spread hate and negativity. Software to combat nefarious deepfakes are currently in development, but only time will reveal the fate of deepfake technology.
[1] Synthetic media: A general term for artificially produced or computerized content; commonly involves AI algorithms.
[2] MIT Sloan School of Management
[3] Variety
[4] Deeptrace
[5] Sentinel
[6] Rolling Stone
[7] Multimedia Forensics
[8] Netizens: Internet citizens